Like father like son: Artificial intelligence is only as racist, xenophobic, and sexist as we are
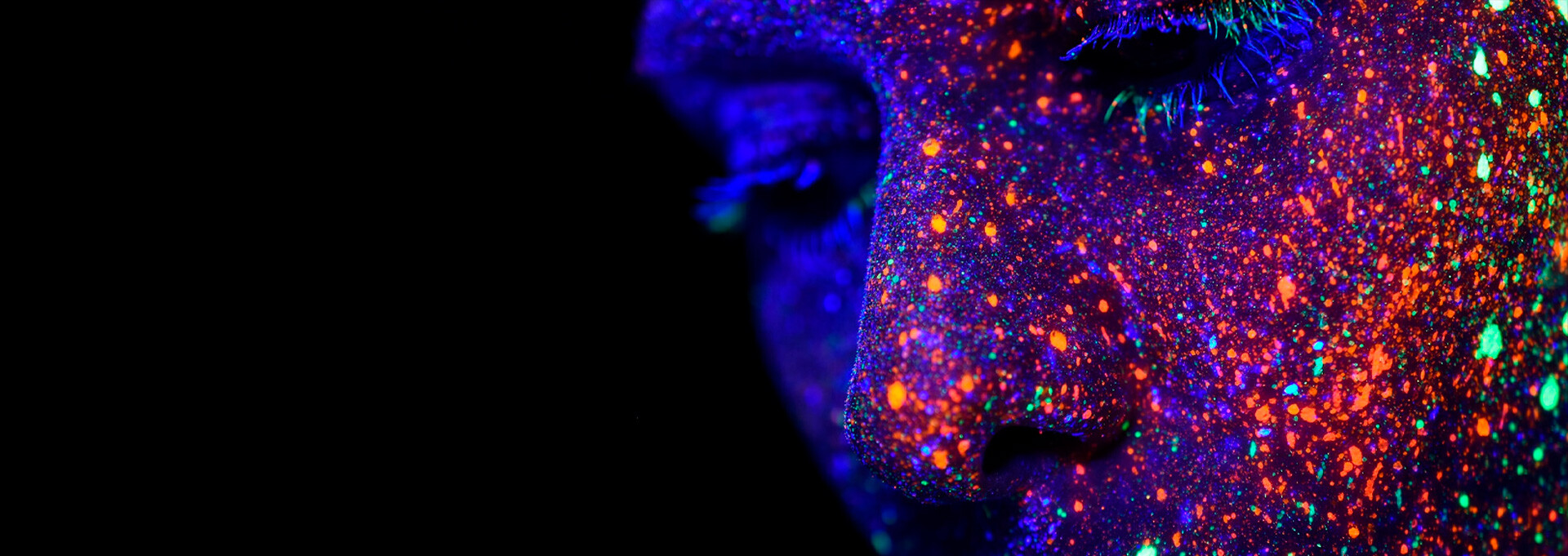
Trying to make the world a better place, artificial intelligence seems to have picked up all the possible flaws and wrongs humanity has been producing for centuries. As a result, we get racist, sexist, and xenophobic AI. A robots' ability to collect and process immense amounts of data comes in handy, but at the same time, it acts like a colossal combine harvester that chews up all it sees. Machines can't learn from mistakes, and people can, so these bugs to the system need to be weeded out before they lead to even bigger trouble.
Machine learning programmes are very appealing — they can comb through incredible amounts of data and make very accurate calculations and predictions for the future based on all that knowledge. This is extensively used in medicine to conclude what drugs and treatment are best suited for individual patients. It can be used in HR to match jobs with candidates or solve other problems that need analysis of massive data arrays.
For example, IBM's Watson computer diagnosed 90% of cancer patients, while human doctors could only identify 50%. On a lighter note, a group of Finnish researchers were able to teach AI to define the music genre based on a person's dance moves . Robots have also learned to trace hackers and predict the future.
However, the fact that AI teachers are ordinary internet users with all their human attitudes and prejudices skews the glowing future of machine learning in the wrong direction. Recently, MIT was asked to take down a dataset which trained AI systems to potentially describe people using racist, misogynistic, and xenophobic vocabulary. The idea for the set was to identify and list depictions in still images automatically. As a result, on many occasions, the system could label women as 'whores' or 'bitches', and Black and Asian people with derogatory language.
According to the study, researchers have come across racial bias in speech recognition systems from Amazon, Apple, Google, IBM and Microsoft — they misidentified white people's words about 19 per cent of the time. In contrast, for black people, it was 35 per cent. Around two per cent of audio recorded by white people was marked as unreadable, and the same was said about 20 per cent of black people. This mishap resulted from insufficient data that was not diverse enough.
Justice system
Another major concern is using imperfect AI to predict crimes or even to give out sentences.
In the legal case against Eric Loomis in Wisconsin, the justice system heavily relied on the Compas algorithm to determine the likelihood of repeat offences, thus sentencing Loomis to six years. His attorneys argued against this ruling as they pointed out the faultiness of the algorithm and its inability to have a more individual approach to every case.
In 2016, ProPublica published an investigation which dealt with a machine learning programme used in court to single out prospective recidivists. The reporters spotted the programme giving out higher risk scores to black people.
The programme's research was, as always, based on the previous incarceration records, a somewhat cavalier approach, since the history of the justice system has proved to be unfair to black Americans. "If computers could accurately predict which defendants were likely to commit new crimes, the criminal justice system could be fairer and more selective about who is incarcerated and for how long," ProPublica wrote. The irony was that instead of providing an impartial approach, the machine learning programmes just took the biases on a global level. As a result, along with judges being prejudiced against African Americans, so were the robots.
Healthcare
Such inadequacy proves to be just as dangerous when it comes to medicine. The study revealed the algorithm forecasting the high-risk care used for over 200 million Americans is racially biased because it relies on faulty metrics.
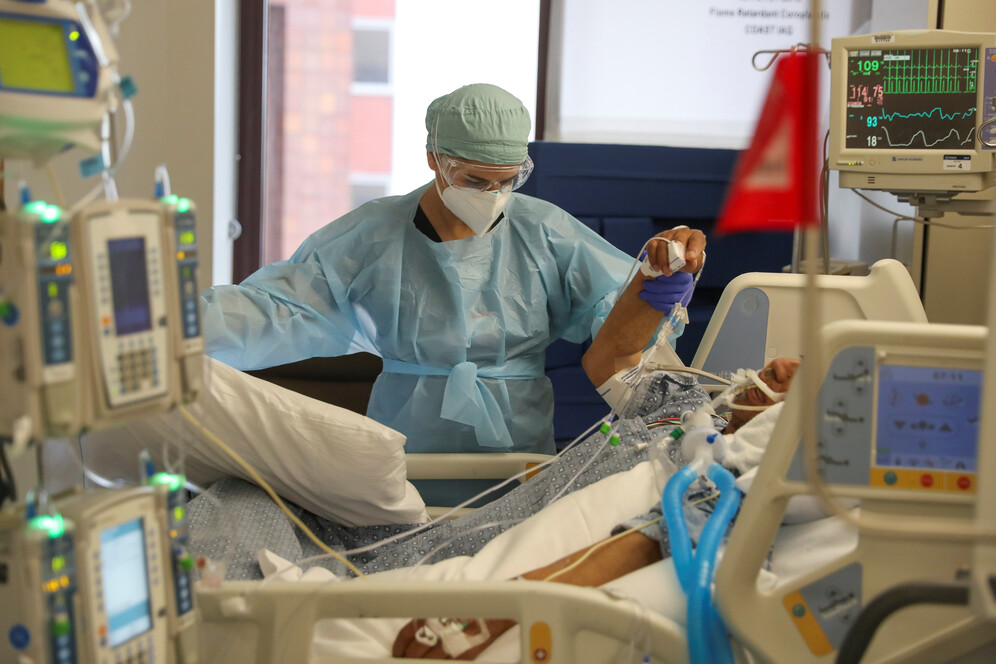
The degree of medical need for every patient was calculated through their medical expenses which proved to be the wrong approach. Due to various social and cultural reasons, black and white people met different medical needs for the same amount of money. While black people would come to the hospital to treat severe chronic diseases like hypertension or diabetes, the whites would show up for non-emergencies, because they could afford an extra visit to the doctor. Because their health care expenses were the same as those of healthier white people, the programme was less likely to flag eligible black patients for high-risk care management. Non-white people have lower incomes which lead to fewer doctor visits. The paper showed black patients have better health outcomes when they have black doctors because of higher levels of trust between doctor and patients. And without the trust, patients are less likely to request extra procedures or treatment and therefore pay for it.
Language
"Many people think machines are not biased," researcher Aylin Caliskan says, "But machines are trained on human data. And humans are biased." In 2017, Caliskan and colleagues published a paper which proves as an AI system teaches itself English, it becomes prejudiced against black Americans and women.
The idea was to teach computers "to talk" to people and understand the slightest nuances of their language. For that, a machine learning programme combed through 840 billion words and looked at how these words appear in sentences. The more frequently two words appear together, the more tied to each other they are in people's everyday speech. Computers learn how to be racist and sexist the way a child does. For example, the word "container" will be most often paired up with "milk" or "liquid".
When it came to people, however, most frequent combinations for Europeans were with "family", "friends", and "happy", while black people were associated with the words that connote unpleasantness. And it's not because the black people are unpleasant, but because bad things are said about them. However, this distinction is not grasped by AI, which in the end yields faulty results.
Such discrepancy may instigate an unjust approach to, for example, recruiting and may hinder the employer from picking the right candidate.
"Let's say a man is applying for a nurse position; he might be found less fit for that position if the machine is just making its own decisions," Aylin Caliskan said. "And this might be the same for a woman applying for a software developer or programmer position. … Almost all of these programmes are not open source, and we're not able to see what's exactly going on. So we have a big responsibility about trying to uncover if they are being unfair or biased," concludes Caliskan.
Immigration and employment
The relationship between AI development and xenophobia is complex. On the one hand, AI can both find and fight xenophobic manifestations online, but on the other hand, it can just as successfully defend them. At the same time, xenophobic attitudes married to strict immigration policy can significantly speed up the deployment of AI systems. Automation and robotisation of industries also create social tension and national intolerance, and with the Trump administration clamping down on immigration, businesses have no other option but to consider replacing the human workforce with machines.
"We live in a world where the friction of xenophobia or the friction of anti-immigration policies actually can lead to technological advancements in an odd way," Illah Nourbakhsh, Professor of Ethics and Computational Technologies at Carnegie Mellon University, told Engadget resource.
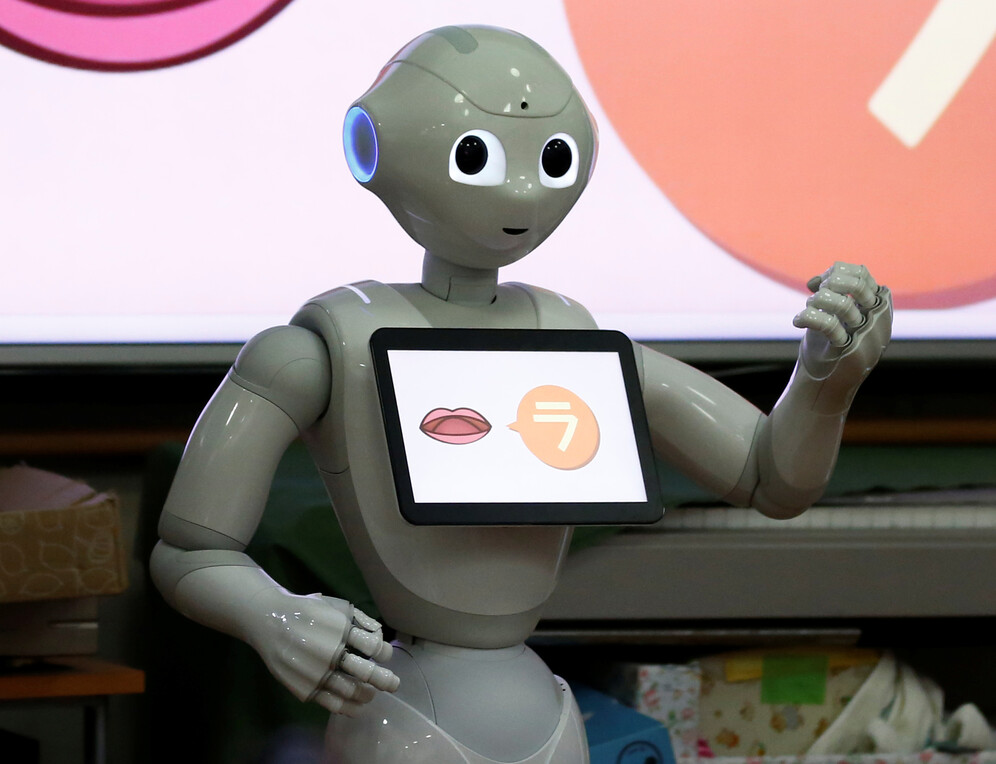
He lists Japan as one of the "good" examples. By 2025, the country will have educated at least 385,000 new healthcare workers to take care of the growing number of seniors in the population.
On other cases, AI can even be instrumental in supporting the existing racist or xenophobic situation. A vivid example is the "virtual border wall" prototype developed by the company owned by former Oculus founder, Palmer Luckey. Equipped with sensors and cameras, the so-called "lettuce" can spot any moving objects, such as an illegal immigrant crossing the US border. The company is hopeful the government will show an interest in the technology given the current attitude towards immigration.
Technological progress aside, such development can lead to mass layoffs and possibly lead to economic collapse.